Community Standards Enforcement Report
In their 5th Community Standards Enforcement Report on how well they enforced their policies from October 2019 to March 2020, Facebook says that they were able to proactively find almost 90% of hate speech, even before it was reported. This is an increase of 24% since the first report in 2018. On Instagram, they increased proactive detection of suicide and self-injury content to 89.7%, up 12 points since the last report.
Additional policy areas that are being monitored are hate speech, adult nudity and sexual activity, violent and graphic content, bullying and harassment, as well as organized hate.
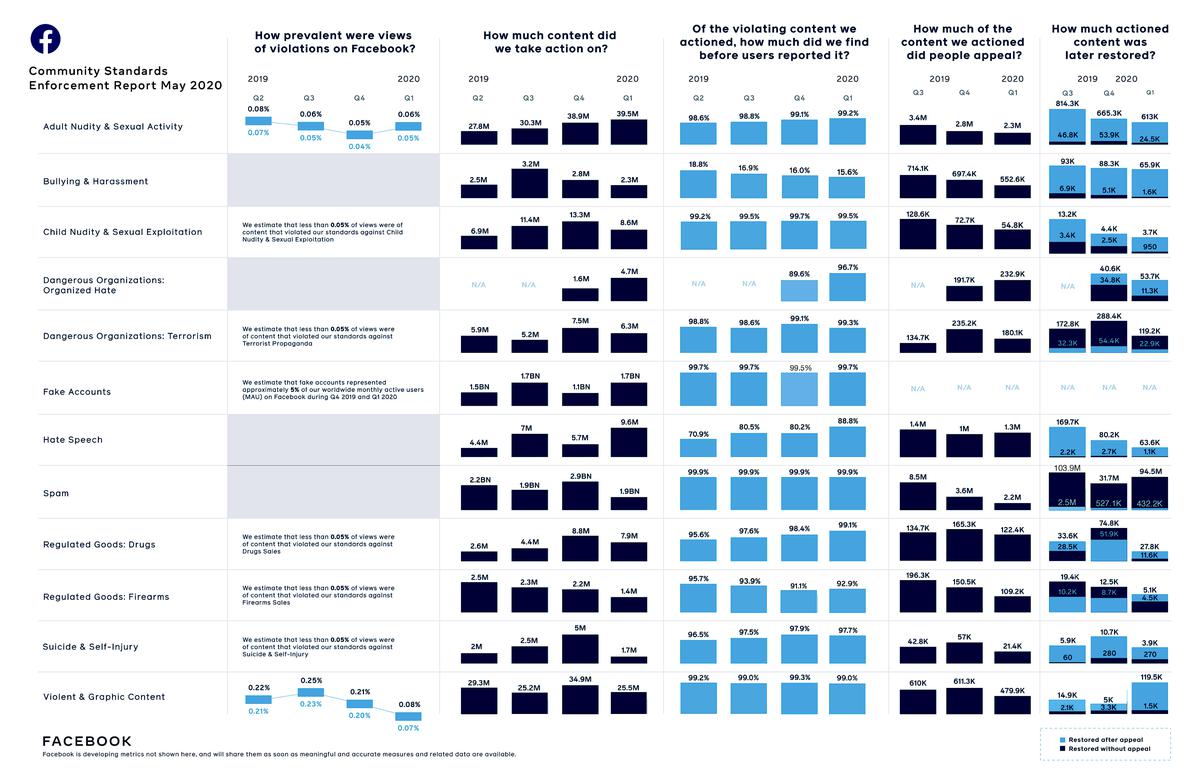
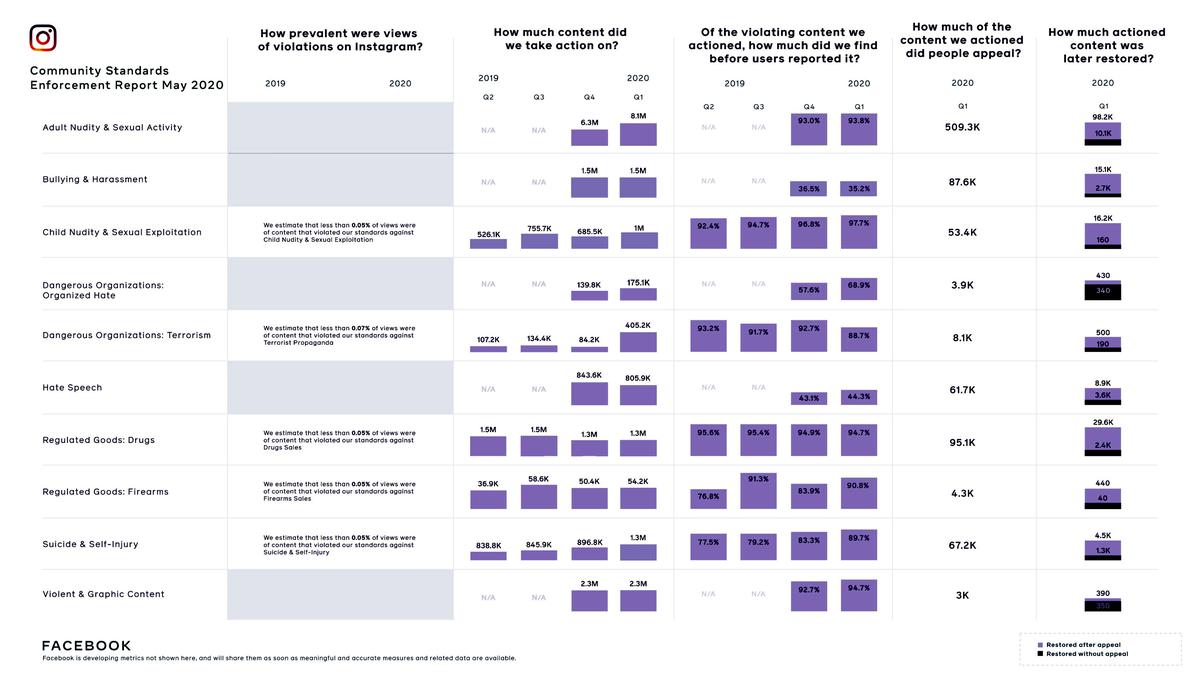
AI and hate speech
Facebook reports that advances in AI have contributed to detecting hate speech through improvements in cross-lingual undersanding as well as whole post or multi-model understanding.
Cross-lingual understanding is the ability to build classifiers that understand the same concept in multiple languages— and learning in one language can improve its performance in others. This is particularly useful for languages that are less common on the internet.
Whole post or multimodal understanding is the ability to train not just on text or images in isolation but to put all of them together to understand the post as a whole. This technology is now used at scale to analyze content.
Facebook has also open sourced the first-ever hateful memes data set, containing more than 10,000 newly created examples of multimodal content (text and images) for an industry-wide challenge to tackle hate. These efforts will spur the broader AI research community to test new methods, compare their work, and benchmark their results in order to accelerate work on detecting multimodal hate speech.
Artificial intelligence (AI) and COVID-19
Although the report does not reflect the full scope of the COVID-19 pandemic, Facebook also shared their progress in two key areas — artificial intelligence and misinformation.
AI is a crucial tool to prevent the spread of misinformation, because it allows the social media giant to leverage and scale the work of the independent fact-checkers who review content on the platform.
SimSearchNet (SSN): When a piece of content has been labeled by independent third-party fact-checking partners as misinformation, SSN can detect near-perfect matches—for example, when someone has tried to alter a piece of content to bypass Facebook systems. SimSearchNet reviews billions of photos every day to find copies of false or misleading information that’s already been debunked.
Image match for ads: New computer vision classifiers help enforce the temporary ban of ads and commerce listings for medical face masks and other products. Because people sometimes modify their ads for these products to try to sneak them past Facebook systems, local feature-based instance matching are also being utilized to find these instances of manipulated media at scale. In many cases, Facebook then takes action proactively — before flagged it has been flagged by anyone. The models based on instance matching results are now auto-rejecting thousands of distinct ads daily with very high accuracy.
Progress on COVID-19 misinformation
In the month of April, Facebook applied warning labels to about 50 million pieces of content related to COVID-19 misinformation, based on around 7500 articles by independent fact-checking partners. 95% of the time, when someone sees content with a warning label, they don’t click through to view it.
Since March 1, Facebook has removed more than 2.5 million pieces of organic content for the sale of masks, hand sanitizers, surface disinfecting wipes and Covid-19 test kits. This has been done using computer vision technology that has been used to find and remove firearm and drug sales.